Satellite-based remote sensing of cloud properties
The satellite image on the left is a composite color full-disk visible image of the Western Hemisphere was captured from NOAA GOES-16 satellite at 1:07 pm EST on Jan. 15, 2017 (the very first image of GOES-16). The “white stuff” in the image are clouds. In fact, at any given time, clouds over about 60% of the surface area of our planet. They play a pivotal role in determining the radiative energy budget of our climate system. On one hand clouds reflect the incoming solar energy back to space which tends to cool the surface. On the other hand, same as greenhouse gases clouds absorb the thermal infrared energy emitted by surface which keeps the surface warm.
A major research area our group has been working on since 2011 is in the satellite-based remote sensing of marine boundary layer (MBL) clouds. MBL clouds cover about 20% of Earth’s surface and play a critical role in modulating the radiative energy budget of our climate system. At present, satellite-based remote sensing is the only means of measuring the properties of MBL clouds, such as the cloud water budget and cloud droplet size, on the global scale. Although operational satellite products from satellite sensors, such as MODIS (Moderate Resolution Imaging Spectroradiometer), have been available for many years, the quality and uncertainties of these products remain largely unknown. For example, even though clouds have highly variable 3-D structures, they are usually assumed to be homogeneous both vertically and horizontally, known as the plane-parallel cloud assumption, in the operational satellite remote sensing algorithms for simplicity. A major branch of our research is focusing on the satellite remote sensing of MBL clouds. More specifically, our research in the direction aims to identify and explain the problems and errors caused by the plane-parallel cloud assumption in MBL remote sensing and explore the novel method for improvement. Since 2011, our group published a series of papers in this direction [Zhang and Platnick, 2011; Zhang et al., 2012; Zhang, 2013; Cho et al., 2015; Zhang et al., 2015; 2016a; Werner et al., 2018a; 2018b; di Noia et al., 2019; Alexandrov et al., 2020]. Together, these papers provide a systematic and insightful understanding of how cloud horizontal and vertical inhomogeneity, and the associated 3-D radiative transfer, influence the satellite-based cloud property retrievals and the potential implications for aerosol-cloud interaction and climate modeling studies
Above-cloud Aerosols: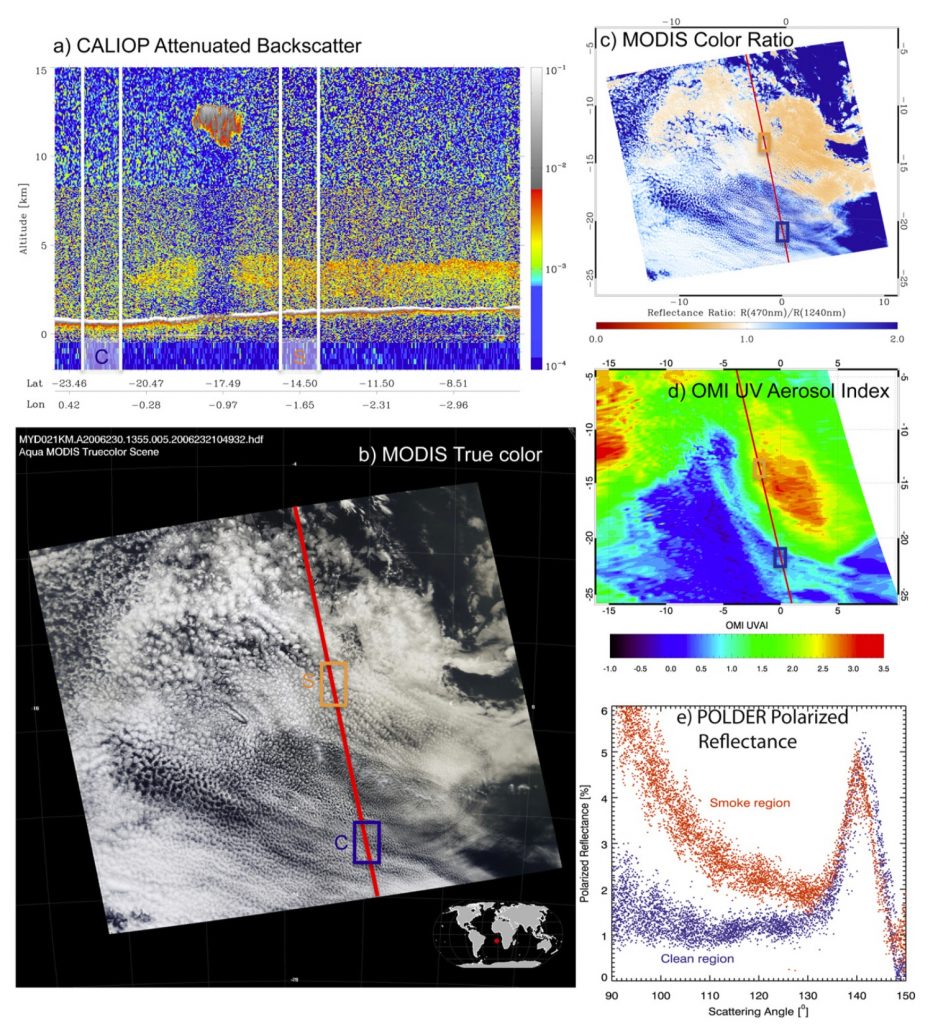
Although aerosols are usually found in boundary layer beneath clouds, they can be elevated during long-range transport and rise above low-level clouds. In fact, above-cloud aerosols are persistently observed in several regions of the globe during certain times of the year. Above-cloud aerosol is an important and interesting component of the climate system for a number of reasons, above all because its shortwave direct radiative effect can differ significantly from that of clear-sky aerosols. The direct radiative effect of aerosols at the top of the atmosphere is strongly dependent on the underlying surface. Over dark surfaces (e.g. ocean surface), the scattering effect of aerosols is generally dominant, leading to cooling effect at top of the atmosphere. In contrast, when aerosols reside above clouds, their absorption effect can be significantly enhanced by cloud reflection, and offset or even exceed the scattering effect of the aerosol leading warming effect at top of the atmosphere. The prevalence and unique radiative effects of above-cloud aerosol make it an important topic for understanding the full complexity of aerosol effects on global radiative energy balance and regional climate. Another important research direction of our group aims to better understand the climatic effects of aerosols that reside above clouds. Since 2013, noteworthy publications from my group in this area include [Yu and Zhang, 2013; Min and Zhang, 2014; Zhang et al., 2014; Meyer et al., 2015; Zhang et al., 2016c; Rajapakshe et al., 2017; Lu et al., 2018]. These studies are among the first to explain the underlying physics that enables the remote sensing of the unique above-cloud aerosols and quantify their impacts on cloud properties and radiative energy budget.
In 2013, Dr. Hongbin Yu and I were invited by the editor of Atmospheric Environments to contribute a review paper on the “Emerging satellite observations of above-cloud aerosols and direct radiative forcing” [Yu and Zhang, 2013]. In this paper, we summarized the current satellite remote sensing techniques for retrieving the properties of above-cloud aerosols. In [Min and Zhang, 2014], my postdoc and I investigated and elucidated the importance of cloud diurnal cycle in regulating the direct radiative effect of above cloud aerosols. In [Zhang et al., 2014], we developed a novel method for computing the direct radiative effect of above-cloud aerosols using NASA’s MODIS and CALIOP datasets. In [Zhang et al., 2016b], this method is applied to 8 years of MODIS and CALIOP data to obtain the direct radiative effect of above-cloud aerosols on a global scale. To our knowledge, this is the first study providing a global perspective on the climate effect of above-cloud aerosols. In Rajapakshe et al., [2017], my student led a study of the height of above-cloud smoke layers with respect to the underlying clouds in the SE Atlantic region using a new space-borne lidar Cloud-Aerosol Transport System (CATS). The key finding is that the smoke layer is much closer to cloud top than previously thought and the implication is that the smoke aerosols can be entrained into the cloud from top and have impacts on the microphysical properties of underlying clouds. Inspired by this finding, we collaborated with a numerical modeling team to investigate the potential pathways through which the entrained smoke aerosols influence the microphysical properties and precipitation efficiency of the underlying clouds. This research led to an important, counterintuitive finding, that is, the above-cloud smoke in the SE Atlantic region have an overall brightening through the so-called Twomey effect rather than the darkening effect through direct interactions with radiation that were found by previous work. This research was published in the Proceedings of the National Academy of Sciences of the United States of America (PNAS) and reported by several online media.
Polarimetric remote sensing of cloud properties
The “traditional” satellite sensors usually measure the intensity (i.e., radiometer) and/or spectral variation (i.e., spectrometer) of light. In comparison, a polarimeter can measure not only the spectral intensity, but also the polarization direction of light and therefore contain more information for remote sensing. Another research direction I have been exploring since around 2014 is the remote sensing of cloud and aerosol properties from satellite-based polarimetric measurements. Multi-directional, multi-wavelength, high-accuracy polarization imager has been identified by the National Research Council’s Earth Sciences Decadal Survey as a key component of NASA’s next generation Earth missions.
Our research along this direction aims to utilize the unique multi-directional, multi-wavelength polarimetric cloud observations to better retrieve cloud properties, in particular to overcome some longstanding issues in radiometric cloud retrievals caused by cloud heterogeneity such as 3-D radiative effects. It is worth mentioning that most of research work in this direction has been carried out by my graduate student under my supervision. My first graduate student, Daniel Miller developed a satellite cloud retrieval simulator based on the combination of polarized radiative transfer model and large-eddy simulation model to simulate the polarimetric, multi-angular, and multi-spectral measurements from the synthetic cloud fields [Miller et al., 2016]. Using this framework, he later led a comparison study to understand how sub-pixel scale cloud inhomogeneity affect the radiometric- and polarimetric-based cloud property retrievals differently [Miller et al., 2018]. These novel research by Dan had helped him won the prestigious NASA Earth System Earth and Space Science Fellowship (NESSF) and later the NASA’s Postdoctoral Program (NPP). Over the last few years, another graduate student of mine, Chamara Rajapakshe, significantly extended Dan’s research. He greatly improved the capability of the satellite cloud retrieval simulator by coupling it with a powerful 3-D vector radiative transfer model developed by our group [Wang et al., 2017; 2019]. In Rajapakshe and Zhang [2020], we used this improved simulator to explore the possibility of utilizing multi-directional polarimetric observations to detect and correct the retrieval bias caused by 3-D radiative effects. The result is very encouraging, and we are now working with collaborators to apply this novel method in real observations. At this moment, Rajapakshe and I are working on two more papers that discuss the different impacts of 3-D radiative effects on radiometric vs. polarimetric cloud property retrievals. It is worth special mentioning that NASA’s Plankton, Aerosol, Cloud and ocean Ecosystem (PACE) mission, which will be launched in 2022-2023 timeframe, will be equipped by two polarimeters (actually one named HARP will be from UMBC’s Dr. Vanderlei Martins’ team). In summary, our main contribution in this area is to develop a state-of-the-art polarimetric cloud property retrieval simulator which has enabled us to reveal some great potentials, as well as weaknesses, of polarimetric observations for cloud remote sensing in comparison with the “traditional” radiometric methods. Recently we become a science team member of the PACE mission. Together with our collaborators, we will be responsible for developing a novel polarimetric-based cloud retrieval product that will be used by the PACE mission and the broad public for many years to come.
Evaluate and improve of low-cloud simulations in climate models
As explained above, low clouds play a critical role in our climate system. Therefore, a faithful simulation of low clouds in the global climate model (GCM) is critical for the projection of future climate and understanding of aerosol-cloud interactions. Unfortunately, it turns out to be an extremely challenging task. Among others, an important reason is that many physical processes in low clouds occur at the spatial scales much smaller than the typical resolution of GCMs, making the simulation of these processes in GCMs highly challenging. One of our recent research directions aims to identify and understand the problems associated with low-cloud simulations in the GCMs and explore pathways for improvements. As the first one of a series of papers, Song et al. [2018b] (led by my postdoc) compared the low-cloud simulations in widely used GCM with satellite observations. We found that the GCM significantly underestimates the low-cloud fraction over the tropical region. More importantly we are able to identify one important reason that has partly caused the low-cloud deficiency in the model, that is, the warm-rain process in the GCM is too frequent that causes low clouds in the model to rain out their water and dissipate too quickly. Later, my postdoc Song led another study that elucidated the importance of considering subgrid cloud variation in the comparison between low-resolution GCM cloud simulations and high-resolution satellite observation [Song et al., 2018a]. Inspired by these two studies, we derived the subgrid variations of cloud microphysical properties over the tropical oceans using satellite observations and use the results to investigate the implications of subgrid cloud variation on the warm-rain parameterization in GCMs [Zhang et al., 2019]. In short, our study elucidated that not only the subgrid variation of cloud water, but the subgrid variation of cloud droplet number concentration and its covariation with cloud water should also be considered in the warm-rain parameterization but are overlooked in most previous studies. Currently, supported by a grant from the DOE, we are further investigating the impacts of subgrid cloud variations on the parametrization of warm rain and thereby the lifetime of low clouds in GCMs.
Dust aerosols: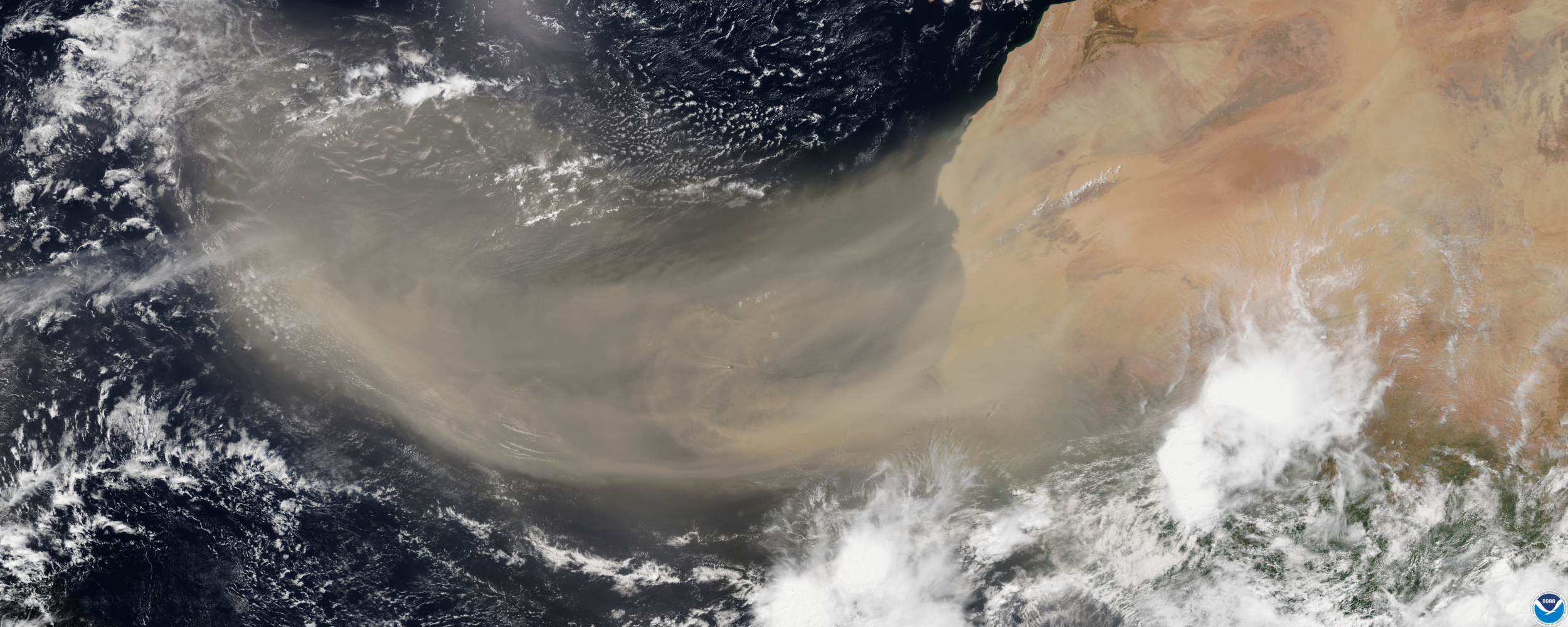
Mineral dust aerosols are the most abundant type of atmospheric aerosols in terms of dry mass. They directly interact with both solar and thermal infrared radiation, known as the direct radiative effect, and thereby influence Earth’s radiative energy budget. Mineral dust aerosols could also influence the life cycle and properties of clouds, by altering the thermal structure of the atmosphere and by acting as cloud condensation nuclei (CCN) and ice nuclei (IN). Dust storms and plumes can degrade air quality and generate adverse impacts on human health. These effects have given dust aerosols an important role in our Earth systems. Together with our collaborators, we aim to use satellite observation to understand the transport and distribution of dust aerosols, their optical and microphysics properties and their impacts on Earth’s radiation and geochemical cycles. Some of our publications in this direction include [Huang et al., 2015; Yu et al., 2015; Zhang et al., 2016c; Song et al., 2018c; Yu et al., 2019]. In a recent study led by my current student Qianqian Song, we derived both shortwave and longwave direct radiative effects of dust aerosols using the combination of satellite observations and radiative transfer simulations. One highly important finding from this study is that the warming effect of dust aerosols in the longwave is significantly (about 30%) and in the opposite sign compared to the cooling effect of dust in the shortwave region. Last year, Qianqian won the prestigious and highly competitive FINESST fellowship from NASA, which will support her to continue her research on dust radiative effects. In addition, our group also received a grant from the CALIPSO-CloudSat program last year to study the radiative signature of dust aerosols in the thermal infrared region. This research will fill an important gap in our observation of dust during nighttime and help us better quantify the net radiative effect of dust aerosols.
Interdisciplinary research on applications of big-data and AI in Earth sciences
In recent years, I am fortunate to team up with a group in the department of Information Systems of UMBC, led by Dr. Jianwu Wang, to work together on some highly interesting and important interdisciplinary research. Dr. Wang is an expert in the areas of “Big Data” and artificial intelligence (AI). Our collaborative research aims to use the latest advances of technology to tackle some long-lasting challenges in the areas of satellite remote sensing, climate modeling and data analytics. Another important objective of this collaboration for me is to introduce the “Big Data” and AI, which are the most vibrant areas of research in recent years, to our physics students. In 2018, we won a NSF grant to launch “Multidisciplinary Research and Education on Big Data + High-Performance Computing + Atmospheric Sciences” (known as the “UMBC Cyber-training”). With this NSF support, we have successfully finished three years of cyber-training which attracted ~50 graduate students from UMBC (5 from the ATPH) and other universities from the U.S. This program has been highlighted many times in UMBC website. Dr. Wang and I were invited by the UMBC IAAC (Interdisciplinary Activities Advisory Committee) to give a talk on our interdisciplinary collaboration experiences. In addition to education and outreach, the cyber-training program has also inspired many interesting research projects. In my view, a few projects could even lead to breakthroughs in satellite remote sensing. For example, one of the cyber-training student teams has been working on dust plume detection from satellite images using AI-based algorithm. The preliminary results are highly encouraging and could later be used in many applications, such as real-time dust event detection and tracking. Another example of our interdisciplinary collaboration is a NASA funded project to develop a novel and user-customizable data processing and analytic infrastructure based on the big-data techniques to aggregate irregular discontinuous pixel-level satellite observations to regular grid-level continuous products. This research could provide the roadmap for the data processing system of future NASA satellite missions. Recently, our interdisciplinary team has won a major grant to “Develop Passive Satellite Cloud Remote Sensing Algorithms using Collocated Observations, Numerical Simulation and Deep Learning” from NASA’s Advancing Collaborative Connections for Earth System Science (ACCESS) Program. One objective of this research is to use the combination of AI and collocated passive-active observations to develop a novel satellite cloud remote sensing algorithm that is hopefully free of the impacts of 3-D radiative transfer effects. This grant will bring over 1.2 million research funding to UMBC for the next three years.